

Siem Jan Koopman
Biography
Siem Jan Koopman is Professor of Econometrics at Vrije Universiteit Amsterdam.
Key publications

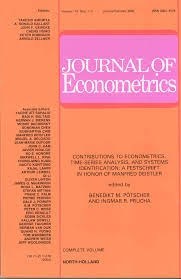















































List of publications
Blasques, F., van Brummelen, J., Gorgi, P. and Koopman, S.J. (2024). Maximum Likelihood Estimation for Non-Stationary Location Models with Mixture of Normal Distributions Journal of Econometrics, 238(1):1--22.
Blasques, F., van Brummelen, J., Gorgi, P. and Koopman, S.J. (2024). A robust Beveridge–Nelson decomposition using a score-driven approach with an application Economics Letters, 236:1--5.
Gorgi, P., Koopman, S.J. and Schaumburg, J. (2024). Vector autoregressions with dynamic factor coefficients and conditionally heteroskedastic errors Journal of Econometrics, :.
Creal, D., Koopman, S.J., Lucas, A. and Zamojski, M. (2024). Observation-driven filtering of time-varying parameters using moment conditions Journal of Econometrics, 238(2):.
Blasques, F., D\textquoterightInnocenzo, E. and Koopman, S.J. (2024). Common and idiosyncratic conditional volatility: Theory and empirical evidence from electricity prices Econometric Reviews, 43(8):638--670.
Blasques, F., Harvey, A.C., Koopman, S.J. and Lucas, A. (2023). Time-Varying Parameters in Econometrics: The editor's foreword Journal of Econometrics, 237(2):.
Gorgi, P. and Koopman, S.J. (2023). Beta observation-driven models with exogenous regressors: A joint analysis of realized correlation and leverage effects Journal of Econometrics, 237(2):1--21.
Winter, J.D., Koopman, S.J. and Hindrayanto, I. (2022). Joint Decomposition of Business and Financial Cycles: Evidence from Eight Advanced Economies* Oxford Bulletin of Economics and Statistics, 84(1):57--79.
Blasques, F., van Brummelen, J., Koopman, S.J. and Lucas, A. (2022). Maximum likelihood estimation for score-driven models Journal of Econometrics, 227(2):325--346.
Blasques, F., Koopman, S.J. and Nientker, M. (2022). A time-varying parameter model for local explosions Journal of Econometrics, 227(1):65--84.
Bennedsen, M., Hillebrand, E. and Koopman, S.J. (2021). Modeling, forecasting, and nowcasting U.S. CO2 emissions using many macroeconomic predictors Energy Economics, 96:1--17.
van de Werve, I., Blasques, F., Koopman, S.J. and Heres Hoogerkamp, M. (2021). Dynamic factor models with clustered loadings: Forecasting education flows using unemployment data International Journal of Forecasting, 37(4):1426--1441.
Li, M. and Koopman, S. (2021). Unobserved components with stochastic volatility: Simulation-based estimation and signal extraction Journal of Applied Econometrics, 36(5):614--627.
Blasques, F., Gorgi, P. and Koopman, S.J. (2021). Missing observations in observation-driven time series models Journal of Econometrics, 221(2):542--568.
Li, M., Koopman, S.J., Lit, R. and Petrova, D. (2020). Long-term forecasting of El Niño events via dynamic factor simulations Journal of Econometrics, 214(1):46--66.
Blasques, F., Koopman, S.J. and Lucas, A. (2020). Nonlinear autoregressive models with optimality properties Econometric Reviews, 39(6):559--578.
Bräuning, F. and Koopman, S.J. (2020). The dynamic factor network model with an application to international trade Journal of Econometrics, 216(2):494--515.
Borowska, A., Hoogerheide, L., Koopman, S.J. and van Dijk, HermanK. (2020). Partially censored posterior for robust and efficient risk evaluation Journal of Econometrics, 217(2):335--355.
Koopman, S.J. and Lit, R. (2019). Forecasting football match results in national league competitions using score-driven time series models International Journal of Forecasting, 35(2):797--809.
Blasques, F., Gorgi, P. and Koopman, S.J. (2019). Accelerating score-driven time series models Journal of Econometrics, 212(2):359--376.
Gorgi, P., Koopman, S.J. and Li, M. (2019). Forecasting economic time series using score-driven dynamic models with mixed-data sampling International Journal of Forecasting, 35(4):1735--1747.
Barra, I., Borowska, A. and Koopman, S.J. (2018). Bayesian dynamic modeling of high-frequency integer price changes Journal of Financial Econometrics, 16(3):384--424.
Koopman, S.J., Lit, R., Lucas, A. and Opschoor, A. (2018). Dynamic discrete copula models for high-frequency stock price changes Journal of Applied Econometrics, 33(7):966--985.
Barra, I., Hoogerheide, L., Koopman, S. and Lucas, A. (2017). Joint Bayesian Analysis of Parameters and States in Nonlinear, Non-Gaussian State Space Models Journal of Applied Econometrics, 32(5):1003--1026.
Calvori, F., Creal, D., Koopman, S.J. and Lucas, A. (2017). Testing for parameter instability across different modeling frameworks Journal of Financial Econometrics, 15(2):223--246.
Schwaab, B., Koopman, S. and Lucas, A. (2017). Global Credit Risk: World, Country and Industry Factors Journal of Applied Econometrics, 32(2):296--317.
Koopman, S. and Mesters, G. (2017). Empirical Bayes Methods for Dynamic Factor Models Review of Economics and Statistics, 99(3):486--498.
Koopman, S., Lit, R. and Lucas, A. (2017). Intraday Stochastic Volatility in Discrete Price Changes: the Dynamic Skellam Model Journal of the American Statistical Association, 112(520):1490--1503.
Blasques, F., Koopman, S., Lasak, K. and Lucas, A. (2016). Rejoinder to the discussion 'In-Sample Confidence Bands and Out-of-Sample Forecast Bands for Time-Varying Parameters in Observation-Driven Models' International Journal of Forecasting, 32(3):893--894.
Blasques, F., Koopman, S., Lucas, A. and Schaumburg, J. (2016). Spillover dynamics for systemic risk measurement using spatial financial time series models Journal of Econometrics, 195(2):211--223.
Vujic, S., Commandeur, J. and Koopman, S. (2016). Intervention time series analysis of crime rates: The case of sentence reform in Virginia Economic Modelling, 57:311--323.
Blasques Albergaria Amaral, F., Koopman, S., Mallee, M. and Zhang, Z. (2016). Weighted Maximum Likelihood for Dynamic Factor Analysis and Forecasting with Mixed Frequency Data Journal of Econometrics, 193(2):405--417.
Blasques, F., Koopman, S., Lasak, K. and Lucas, A. (2016). In-Sample Confidence Bands and Out-of-Sample Forecast Bands for Time-Varying Parameters in Observation Driven Models International Journal of Forecasting, 32(3):875--887.
Mesters, G., Koopman, S. and Ooms, M. (2016). Monte Carlo Maximum Likelihood Estimation for Generalized Long-Memory Time Series Models Econometric Reviews, 35(4):659--687.
Hindrayanto, A., Koopman, S. and de Winter, J. (2016). Forecasting and nowcasting economic growth in the euro area using factor models International Journal of Forecasting, 32(4):1284--1305.
Nucera, F., Schwaab, B., Koopman, S. and Lucas, A. (2016). The Information in Systemic Risk Rankings Journal of Empirical Finance, 38A(September):461--475.
Koopman, S., Lucas, A. and Scharth, M. (2016). Predicting time-varying parameters with parameter-driven and observation-driven models Review of Economics and Statistics, 98(1):97--110.
Galati, E., Hindrayanto, A., Koopman, S. and Vlekke, M. (2016). Measuring Financial Cycles in a Model-Based Analysis: Empirical Evidence for the United States and the Euro Area Economics Letters, 145:83--87.
Jungbacker, B. and Koopman, S. (2015). Likelihood-based Dynamic Factor Analysis for Measurement and Forecasting Econometrics Journal, 18(2):C1--C21.
Koopman, S., Lucas, A. and Scharth, M. (2015). Numerically Accelerated Importance Sampling for Nonlinear Non-Gaussian State Space Models Journal of Business and Economic Statistics, 33(1):114--127.
Blasques, F., Koopman, S. and Lucas, A. (2015). Information Theoretic Optimality of Observation Driven Time Series Models Biometrika, 102(2):325--343.
Koopman, S., Lucas, A. and Schwaab, B. (2014). Nowcasting and forecasting global financial sector stress and credit market dislocation International Journal of Forecasting, 30(3):741--758.
Janus, P., Koopman, S. and Lucas, A. (2014). Long memory dynamics for multivariate dependence under heavy tails Journal of Empirical Finance, 29(December):187--206.
Creal, D., Schwaab, B., Koopman, S. and Lucas, A. (2014). Observation Driven Mixed-Measurement Dynamic Factor Models with an Application to Credit Risk Review of Economics and Statistics, 96(5):898--915.
Mesters, G. and Koopman, S. (2014). Generalized Dynamic Panel Data Models with Random Effects for Cross-Section and Time Journal of Econometrics, 180(2):127--140.
Jungbacker, B., Koopman, S. and van der Wel, M. (2014). Smooth Dynamic Factor Analysis with Application to the U.S. Term Structure of Interest Rates Journal of Applied Econometrics, 29(1):65--90.
Brauning, F. and Koopman, S. (2014). Forecasting Macroeconomic Variables using Collapsed Dynamic Factor Analysis International Journal of Forecasting, 30(3):572--584.
Dijk, D.van, Koopman, S., van der Wel, M. and Wright, J. (2014). Forecasting Interest Rates with Shifting Endpoints Journal of Applied Econometrics, 29(5):693--712.
Koopman, S. and van der Wel, M. (2013). Forecasting the U.S. Term Structure of Interest Rates using a Macroeconomic Smooth Dynamic Factor Model International Journal of Forecasting, 29(4):676--694.
Koopman, S. and Scharth, M. (2013). The Analysis of Stochastic Volatility in the Presence of Daily Realised Measures Journal of Financial Econometrics, 11(1):76--115.
Creal, D., Koopman, S. and Lucas, A. (2013). General Autoregressive Score Models with Applications Journal of Applied Econometrics, 28(5):777--795.
Hindrayanto, A., Aston, J., Koopman, S. and Ooms, M. (2013). Modeling trigonometric seasonal components for monthly economic time series Applied Economics, 45(21):3024--3034.
Bos, C., Janus, P. and Koopman, S. (2012). Spot Variance Path Estimation and its Application to High Frequency Jump Testing Journal of Financial Econometrics, 10(2):354--389.
Koopman, S., Lucas, A. and Schwaab, B. (2012). Dynamic Factor Models With Macro, Frailty and Industry Effects for U.S. Default Counts: The Credit Crisis of 2008 Journal of Business and Economic Statistics, 30(4):521--532.
Koopman, S., Lucas, A. and Schwaab, B. (2011). Modeling frailty correlated defaults using many macroeconomic covariates Journal of Econometrics, 162(2):312--325.
Creal, D., Koopman, S. and Lucas, A. (2011). A dynamic multivariate heavy-tailed model for time-varying volatilities and correlations Journal of Business and Economic Statistics, 29(4):552--563.
Jungbacker, B., Koopman, S. and van der Wel, M. (2011). Maximum likelihood estimation for dynamic factor models with missing data Journal of Economic Dynamics and Control, 35(8):1358--1368.
Koopman, S. and Wong, S. (2011). Kalman filtering and smoothing for model-based signal extraction that depend on time-varying spectra Journal of Forecasting, 30(1):147--167.
Koopman, S. and Ooms, M. (2010). Discussion of `Exponentionally Weighted Methods for Forecasting Intraday Time Series with Multiple Seasonal Cycles -- James W. Taylor' [Review of: Exponentionally Weighted Methods for Forecasting Intraday Time Series with Multiple Seasonal Cycles] International Journal of Forecasting, 26:627--651.
Koopman, S. and Creal, D. (2010). Extracting a robust U.S. business cycle using a time-varying multivariate model-based bandpass filter Journal of Applied Econometrics, 25:695--719.
Koopman, S., Mallee, M. and van der Wel, M. (2010). Analyzing the term structure of interest rates using the dynamic Nelson-Siegel model with time-varying parameters Journal of Business and Economic Statistics, 28:329--343.
Koopman, S. (2010). Discussion of `Particle Markov chain Monte Carlo methods – C. Andrieu, A. Doucet and R. Holenstein{\textquoteright} [Review of: Particle Markov chain Monte Carlo methods] Journal of the Royal Statistical Society. Series B. Statistical Methodology, 72:269--342.
Koopman, S., Shephard, N. and Creal, D. (2009). Testing the assumptions behind importance sampling Journal of Econometrics, 149:2--11.
Koopman, S., Kraeussl, R., Lucas, A. and Monteiro, A. (2009). Credit cycles and macro fundamentals Journal of Empirical Finance, 16:42--54.
Koopman, S., Ooms, M. and Hindrayanto, A. (2009). Periodic unobserved cycles in seasonal time series with an application to U.S. unemployment Oxford Bulletin of Economics and Statistics, 71:683--713.
Koopman, S., Lucas, A. and Monteiro, A. (2008). The Multi-state Latent Factor Intensity Model for Credit Rating Transitions Journal of Econometrics, 142:399--424.
Koopman, S., Dordonnat, V. and Ooms, M. (2008). An Hourly Periodic State Space Model for Modelling French National Electricity Load International Journal of Forecasting, 24:566--587.
Koopman, S. and Valle a Azevedo, J. (2008). Measuring Synchronisation and Convergence of Business Cycles in Eurozone, UK and US Oxford Bulletin of Economics and Statistics, 70(1):23--51.
Koopman, S. and Lucas, A. (2008). A Non-Gaussian Panel Time series Model for Estimating and Decomposing Default Risk Journal of Business and Economic Statistics, 26(4):510--525.
Ooms, M., Koopman, S. and Carnero, A. (2007). Periodic Seasonal Reg-ARFIMA-GARCH Models for Daily Electricity Spot Prices Journal of the American Statistical Association, 102(477):16--27.
Jungbacker, B. and Koopman, S. (2007). Monte Carlo estimation for nonlinear non-Gaussian state space models Biometrika, 94:827--839.
Menkveld, A., Koopman, S. and Lucas, A. (2007). Modelling Round-the-Clock Price Discovery for Cross-Listed Stocks using State Space Methods Journal of Business and Economic Statistics, 25(2):213--255.
Jungbacker, B. and Koopman, S. (2006). Monte Carlo likelihood estimation for three multivariate stochastic volatility models Econometric Reviews, 25(2-3):385--408.
Azevedo, J., Koopman, S. and Rua, A. (2006). Tracking the business cycle of the Euro area: A multivariate model-based band-pass filter Journal of Business and Economic Statistics, 24(3):278--290.
Aston, J. and Koopman, S. (2006). A non-Gaussian generalisation of the Airline model for robust Seasonal Adjustment Journal of Forecasting, 25(5):325--349.
Koopman, S., Jungbacker, B. and Hol, E. (2005). Forecasting daily variability of the S&P 100 stock index using historical, realised and implied volatility measurements Journal of Empirical Finance, 12(3):445--475.
Koopman, S. and Lucas, A. (2005). Business and Default Cycles for Credit Risk Journal of Applied Econometrics, 20:311--323.
Koopman, S., Lucas, A. and Klaassen, P. (2005). Empirical Credit Cycles and Capital Buffer Formation Journal of Banking and Finance, 29:3159--3179.
Koopman, S. and Luginbuhl, R. (2004). Convergence in European GDP Series Journal of Applied Econometrics, 19(5):611--636.
Koopman, S. and Lee, K. (2004). Estimating stochastic volatility models: a comparison of two importance samplers Studies in Nonlinear Dynamics and Econometrics, 8(2):1--22.
Koopman, S. and Bos, C. (2004). State space models with a common stochastic variance Journal of Business and Economic Statistics, 22(3):346--357.
Koopman, S. and Harvey, A. (2003). Computing Observation Weights for Signal Extraction and Filtering Journal of Economic Dynamics and Control, 27(7):1317--1333.
Koopman, S. and Hol Uspensky, E. (2002). The Stochastic Volatility in Mean Model: Empirical evidence from international stock markets Journal of Applied Econometrics, 17:667--689.
Koopman, S. and Franses, P.H. (2002). Constructing seasonally adjusted data with time-varying confidence intervals Oxford Bulletin of Economics and Statistics, 64(5):509--526.
Koopman, S. and Durbin, J. (2002). A simple and efficient smoother for state space time series analysis Biometrika, 89(3):603--616.
Harvey, A. and Koopman, S. (2000). Signal Extraction and the Formulation of Unobserved Components Models Econometrics Journal, 3:84--107.
Koopman, S. and Durbin, J. (2000). Time series analysis of non-Gaussian observations based on state space models from both classical and Bayesian perspectives Journal of the Royal Statistical Society. Series B. Statistical Methodology, 62:3--56.
Koopman, S., Shephard, N. and Doornik, J. (1999). Statistical algorithms for models in state space using SsPack 2.2 Journal of Econometrics, (2):113--166.
Sandmann, G. and Koopman, S.J. (1998). Estimation of stochastic volatility models via Monte Carlo maximum likelihood Journal of Econometrics, 87(2):271--301.
Harvey, A., Koopman, S.J. and Riani, M. (1997). The modeling and seasonal adjustment of weekly observations Journal of Business and Economic Statistics, 15(3):354--368.
Atkinson, A.C., Koopman, S.J. and Shephard, N. (1997). Detecting shocks: Outliers and breaks in time series Journal of Econometrics, 80(2):387--422.
Koopman, S.J. (1997). Exact initial kalman filtering and smoothing for nonstationary time series models Journal of the American Statistical Association, 92(440):1630--1638.
Durbin, J. and Koopman, S.J. (1997). Monte Carlo maximum likelihood estimation for non-Gaussian state space models Biometrika, 84(3):669--684.
Harvey, A. and Koopman, S.J. (1993). Forecasting hourly electricity demand using time–varying splines Journal of the American Statistical Association, 88(424):1228--1236.
Koopman, S.J. (1993). Disturbance smoother for state space models Biometrika, 80(1):117--126.
Harvey, AndrewC. and Koopman, S.J. (1992). Diagnostic checking of unobserved- components time series models Journal of Business and Economic Statistics, 10(4):377--389.
Koopman, S.J. (1992). Miscellanea: Exact score for time series models in state space form Biometrika, 79(4):823--826.